Mastering Labeling Training Data: A Key Component in AI Success
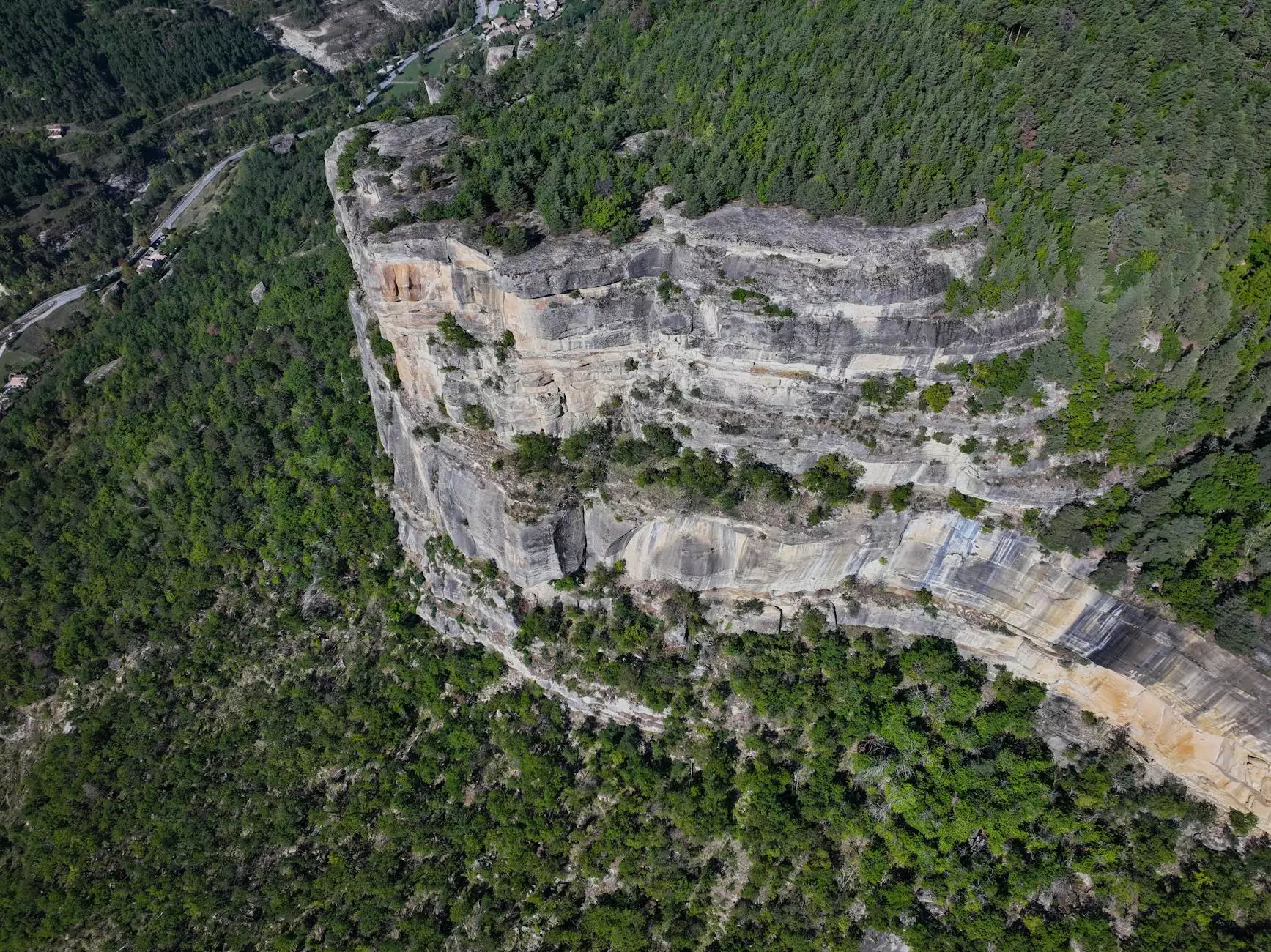
The process of labeling training data is fundamental in the vast landscape of artificial intelligence (AI) and machine learning (ML). At the heart of every successful AI model lies data that has been meticulously annotated, categorized, and organized. In this in-depth article, we will explore the essential role of labeling training data, the methods involved, the challenges faced, and how Keylabs.ai provides unparalleled support in this crucial arena.
Understanding the Basics of Data Annotation
Data annotation is the process of labeling data to make it meaningful and usable for machine learning algorithms. This involves categorizing images, text, audio, and video data so that AI systems can learn from the information efficiently. Proper labeling training data ensures that the AI models are trained to recognize patterns, make predictions, and improve over time.
Types of Data to be Annotated
- Image Data: Labeling can include bounding boxes, polygons, and points to identify objects within images.
- Text Data: This involves tagging entities, sentiment analysis, and categorization of text documents.
- Audio Data: Labels can denote different sounds, transcriptions, or specific audio events.
- Video Data: Annotations can include activity detection, object tracking, and scene recognition.
The Importance of Labeling Training Data in Machine Learning
In the realm of AI, the adage "garbage in, garbage out" holds true. The quality of your AI model is directly proportional to the quality of your training data. Here are several reasons why labeling training data is vital:
1. Enhancing Model Accuracy
Accurate labeling results in a higher quality dataset, which translates to enhanced model accuracy. When the data fed to the machine learning algorithms is labeled correctly, the models can learn better and produce more reliable predictions.
2. Facilitating Effective Training
Training machine learning models requires vast amounts of data. Without proper labels, the data would be meaningless. Proper annotation helps create a rich training dataset that supports learning.
3. Enabling Supervised Learning
Supervised learning heavily relies on labeled data to teach models. The labels serve as the correct answers that the models aspire to achieve, guiding their learning process effectively.
4. Boosting Scalability of AI Models
With clear and consistent labeling practices, organizations can scale their AI models more efficiently. As models are exposed to varied and extensive datasets, they become adept at generalizing in different scenarios.
Challenges in Labeling Training Data
While labeling training data is crucial, it is not without its challenges:
1. Time-Consuming Process
The process of labeling training data can be highly time-consuming, especially when vast datasets are involved. Human annotators must painstakingly go through each piece of data, making the process slow and labor-intensive.
2. Subjectivity in Labels
Different annotators may interpret and label data differently. This subjectivity can lead to inconsistencies within the dataset, negatively impacting model performance.
3. Complexity of Data Types
As data types grow more complex, so do the challenges in labeling them. For instance, annotating a video for object tracking involves a distinct skill set compared to labeling textual sentiment.
4. Requirement for Domain Expertise
Some datasets necessitate domain-specific knowledge for effective labeling. For example, medical images must be annotated by specialists who understand the nuances of the field.
Keylabs.ai: Your Partner in Labeling Training Data
The data annotation landscape is ever-changing, making it imperative to have a robust platform that can adapt to these dynamics. Keylabs.ai positions itself as a leader in this domain, offering innovative tools and solutions to facilitate the seamless annotation of data.
Benefits of Using Keylabs.ai
- High-Quality Annotations: Keylabs.ai provides tools that enable precise and high-quality data annotations, ensuring the best possible training data for AI models.
- User-Friendly Interface: The platform is designed for ease of use, making it accessible for users with varying levels of technical knowledge.
- Scalability: Whether you're handling small datasets or massive volumes of data, Keylabs.ai's infrastructure can scale accordingly.
- Expert Team: Keylabs.ai employs a team of skilled annotators who can address even the most complex labeling tasks across various domains.
- Custom Solutions: The platform offers tailor-made solutions to meet the unique requirements of different industries.
Conclusion: The Future of Labeling Training Data
The journey of labeling training data is not just about creating datasets; it's about laying the foundation for smarter, more efficient AI models. As technology evolves, the methods and tools for data annotation will continue to advance. Organizations that prioritize high-quality, accurate labeling of their training data will undoubtedly lead the charge into the future of AI.
Embracing platforms like Keylabs.ai will empower businesses to harness the full potential of their data, ensuring that their machine learning models are not only functional but also groundbreaking in their capabilities. As we progress into an era where AI shapes our daily lives, the significance of labeling training data will only continue to grow.